Machine Learning and Decision Management:
A standards-based approach. Edson Tirelli and Matteo Mortari, Red Hat
DecisionCAMP Day 1 morning sessions continue with Edson Tirelli and Mateo Mortari presenting on the integration of machine learning and decision management to address predictive decision automation. The problem to date is that integrating machine learning into business automation (either process or decision) has required proprietary interfaces and APIs, although there is an existing standard (PMML, Predictive Model Markup Language) for specifying and exchanging many types of executable machine learning models. The entry of the DMN standard provides a potential bridge between PMML and both BPMN and CMMN, allowing for an end-to-end standards-based representation for cases, processes, decisions and predictive models.

They gave a demo of how they have implemented this using RedHat decision and process engines along with open source tools Prometheus and Grafana, with a credit card dispute use case that uses BPMN, DMN and PMML to model the process and decisions. They started with a standard use of BPMN and DMN, where the DMN decision tables and graphs calculate the risk factors of the dispute and the customer, and make a decision on whether or not the dispute process can be automated. They added a predictive model for better calculation of the risk factors, positioning this in the DMN DRD as a business knowledge model that can then drive the decision model instead of a hard-coded decision table.
They finished their demo by importing the same PMML and DMN models in the Trisotech modeler to show interoperability of the integrated model types, with the predictive models providing knowledge sources for the decision models.
Coming from the process side, this is really exciting: we’re already seeing a lot of proprietary plug-ins and APIs to add machine learning to business processes, but this goes beyond that to allow standards-based tools to be plugged together easily. There’s still obviously work to be done to make this a seamless integration, but the idea that it can be all standards-based is pretty significant.
FEEL, Is It Really Friendly Enough? Daniel Schmitz-Hübsch and Ulrich Striffler, Materna
Materna has a number of implementation projects (mostly German government) that involve decision automation, where logic is modeled by business users and require that the decision justification be able to be explained to all users for transparency of decision automation. They use both decision tables and FEEL — decision tables are easier for business users to understand, but can’t represent everything — and some of the early adopters are using DMN. Given that most requirements are documented by business users in natural language, there are some obstacles to moving that initial representation to DMN instead.
Having the business users model the details of decisions in FEEL is the biggest issue: basically, you’re asking business people to write code in a script language, with the added twist that in their case, the business users are not native English speakers but the FEEL keywords are in English. In my experience, it’s hard enough to get business people to create syntactically-correct visual models in BPMN, moving to a scripting language would be a daunting task, and doing that in a foreign language would make most business people’s heads explode in frustration.
They are trying some different approaches for dealing with this: allowing the users to read and write the logic in their native natural language (German), or replacing some FEEL elements (text statements) with graphical representations. They believe that this is a good starting point for a discussion on making FEEL a bit friendlier for business users, especially those whose native language is not English.
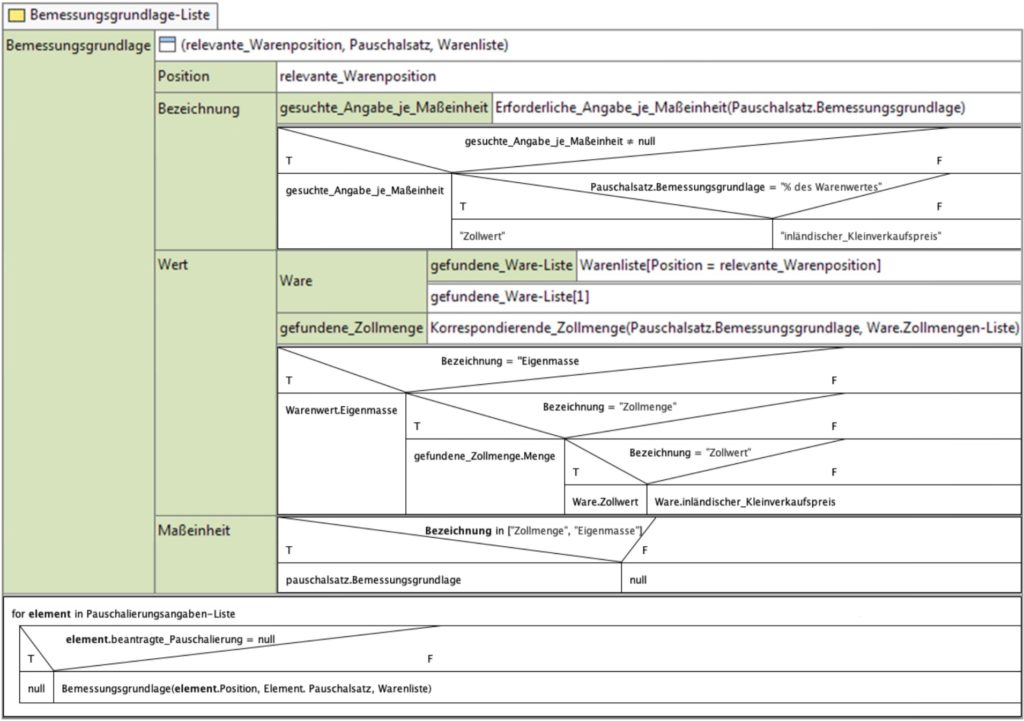
Good closing discussion on the use of different tools for different levels of people doing the modeling.